What Does AI Transparency Look Like? It Depends on Your Industry
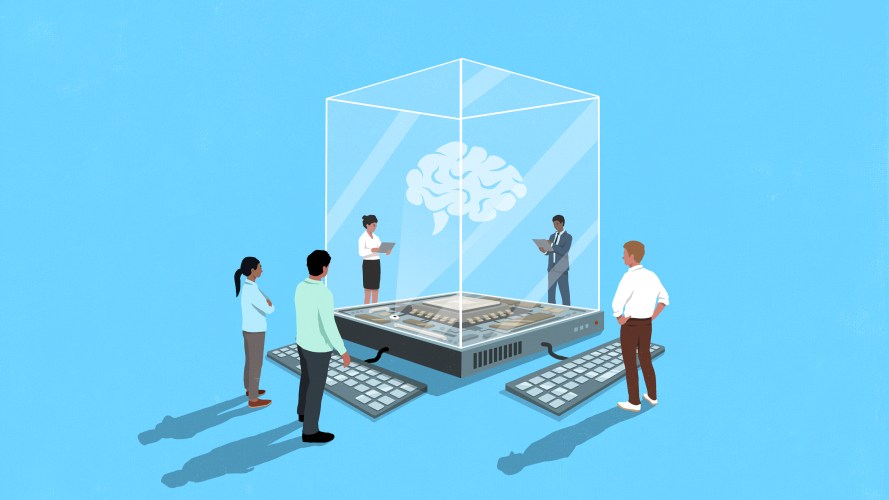
We trust generative AI algorithms with important decisions, but know little about how they work.

Lisa Lee
If you measured it today, how would your business score on AI transparency? Whether you’re using artificial intelligence (AI) to recommend products, develop drugs, or screen job applicants, you’re entrusting it with data without fully understanding how it makes decisions. How can you better understand the logic behind the AI black box, and do a better job of disclosing when — and how — AI is being used?
This lack of transparency threatens privacy and security, and fosters uncompensated use of copyrighted content, according to Senate testimony by Ramayya Krishnan, dean of Carnegie Mellon University’s Heinz College of Information Systems and Public Policy. Still, you can take steps today to make sure you’re being open about how your company is using AI.
Develop ethical generative AI from the get-go
Building trustworthy generative AI requires a firm foundation at the inception of AI development. It helps to follow a framework. These are our guidelines for responsible development.



‘Companies treat their LLMs as trade secrets’
Transparency in AI has always been important, but came to the fore with the October publication of the Foundation Model Transparency Index. The research, led by Stanford University, found all 10 major foundation model companies, including Google and OpenAI, were “lacking” in transparency. And that’s being charitable. When evaluated across 100 aspects of transparency, including the use of copyrighted data and harmful data filtration, on a 100-point scale, the highest-scoring company received a 54.
Large language models (LLMs) are, by their very nature, opaque. Their scale and complexity make it hard to completely understand their decision-making process. In fact, because these models calculate probabilities rather than certainties, you can ask an LLM the same question 10 times and get 10 different responses. At the same time, according to the Prompt Engineering Institute, “companies treat their LLMs as trade secrets, disclosing only limited information about model architecture, training data, and decision-making processes. This opacity prevents independent auditing of the systems for biases, flaws, or ethical issues.”
There’s not much independent auditing happening, anyway. It’s a new practice that, save for outliers like New York City, isn’t mandated by any local, state, or federal authority. But you don’t need to wait for a mandate to implement transparency protocols of your own. Here are some transparency in AI issues that various industries are facing, and tips on how to address them.
Transparency in ecommerce product recommendations
Generative AI can enhance virtually every facet of ecommerce, from personalized customer journeys to curated product recommendations. But where there are consumers, there’d better be data transparency.
Scenario: A clothing company offers AI-based product recommendations but doesn’t clarify how its customers’ personal data is used to make those recommendations, causing a consumer backlash.
Transparency techniques: Establish and communicate clear data usage policies, and be open about why your system recommends what it does. Then, measure, measure, measure. Poll customers both directly and indirectly via service agents that resolve inquiries. It’s important to survey customers across all channels to ensure complaint volume is trending in the right direction.
Implementation techniques: Deploy tools that let customers see and control the data that influences AI recommendations. Use customer education campaigns to communicate your customer data use guidelines and the benefits of personalized recommendations.
Regulated industries have a bigger burden
Companies in regulated industries operate under a different set of rules. Think HIPAA in healthcare and customer protection in financial services. Due to the stringent regulations that govern these sectors, demonstrating transparency in AI is all the more important.
Scenario: A pharmaceutical company uses AI for drug development but struggles to articulate AI’s role in the research, raising concerns about validation and safety.
Transparency techniques: Explain the role of AI in clinical trial data and decision-making processes. Meticulously validate AI-derived findings. Develop a set of AI transparency and compliance protocols tailored to meet regulatory requirements and patient safety concerns. (For example, clearly explain what patient data will and won’t be used for, such as providing care and developing drugs vs. marketing.) Give patients the right to opt out, request all data that’s been used, and learn how it was used.
Implementation techniques: Use traceability systems to better demonstrate AI’s role in the research and development processes. Meet with regulatory bodies early in AI development to ensure alignment and build trust.
Looking for more AI success tips?
Whether you’re just starting out with AI or already innovating, this guide will help you strategize effectively, embrace new possibilities, and answer important questions about the benefits of AI.



Trusting AI to make hiring decisions
More than 60% of companies in a 2022 survey said they use AI to automatically filter out unqualified job applicants. What does “unqualified” mean? It’s hard to say, and that’s probably why most people are wary of AI’s use in hiring decisions.
Scenario: A company’s applicant-screening AI is criticized for serving up homogenous talent pools, raising questions among hiring managers and applicants about the data sets and algorithms used.
Transparency techniques: Publish the model’s decision-making framework, both in the job posting and in the application process. Disclose how the technology is being used and give applicants the option to opt out. Perform regular bias audits to foster transparency and trust. Establish a transparency framework that documents the model’s design, data sources, and oversight mechanisms.
Implementation techniques: Develop an audit trail system to track decision-making in AI-influenced hiring. Ask diverse stakeholders to review and provide input on AI hiring practices as part of a broader effort to ensure equal opportunity in hiring.
Don’t forget to be human
There are other things to consider regarding transparency in AI. For starters, don’t assume that users understand the information you’re providing.
“Transparency does not always equate to understanding,” said William Dressler, director of data strategy at Salesforce. Providing raw data, for example, won’t help non-techies glean meaningful insights about AI or reassure them that the AI is safe and unbiased. When sharing information about how and why your AI systems do what they do, be as clear and plain-spoken as possible.
Transparency is just one component of building trusted AI systems that are safe, reliable, and as free from bias as possible.
Building these systems “involves not only showing the inner workings of AI models but also demonstrating their reliability, fairness, and alignment with human values in practice,” said Dressler. He also advises not to over-index on transparency at the expense of other critical considerations like ethical design, robustness, and privacy.
Finally, decide what you need to be transparent about. The Foundation Model Transparency Index measures openness using 100 criteria. Most companies don’t need to share information across each of those dimensions, as too much information can lead to confusion and indecision, said Dressler.
“Ask yourself, are you overwhelming users with data and making it harder for them to trust AI because they can’t process all the information?”